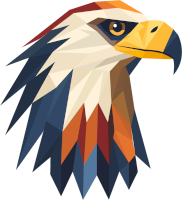
ذكاء الأعمال كخدمة
جرب خدمة PlusClouds إيجلت واكتشف فرص وعملاء محتملين عالي الجودة في مجال الأعمال بين الشركات بدعم الذكاء الاصطناعي
In recent years, artificial intelligence (AI) and machine learning (ML) have begun to revolutionize the real estate sector in areas such as price forecasting, demand analysis, and portfolio management. However, these technologies not only interpret current data but also hold promise in predicting critical scenarios for the future. One of these is real estate bubbles: situations of financial crisis where prices artificially rise by deviating from the supply-demand balance and then burst suddenly. So, can artificial intelligence really predict these bubbles? This is the question we are pursuing.
A real estate bubble is formed when housing prices rise artificially, disconnected from economic fundamentals. This increase is generally fueled by factors such as consumer behavior, speculative investment, cheap credit, and insufficient regulation. At some point, prices become unsustainable, leading to sudden drops ("bursts"). The 2008 crisis is a striking example of this. The ability to detect such scenarios in advance could make a significant difference for investors and policymakers.
Many economic crises could not be predicted using traditional economic models. These models typically rely on linear assumptions, work with static data, and ignore the complex relationships among all variables. However, housing bubbles arise from the interplay of social psychology, behavioral economics, and financial architecture. Therefore, more complex, multidimensional, and data-intensive analyses were needed. It is at this point that artificial intelligence comes into play.
The most significant difference of AI is its ability to recognize relationships, correlations, and patterns among a multitude of complex variables. This skill can be utilized to identify "anomalies" in the real estate market.
Machine learning algorithms can analyze changes in housing prices over time, identifying deviations from the average (outlier situations). These deviations can be examined to determine whether they signal calm increases or potential bursts.
Models can be trained using data from crises like 2008 through supervised learning. In other words, the model is fed with examples such as "these data indicate the presence of a bubble." Similar signals can be sought in current markets.
Considering that bubbles do not always appear the same, unsupervised learning may be more flexible. For instance, abnormal deviations in the ratios between housing prices and rental yields could signal potential bubbles.
(The graph shows mortgage default rates between 2004 and 2010.
The areas marked with red circles are points that could be identified as "abnormal increases" by AI.
Such early signals could indicate the approaching collapse of a market.)
The 2008 crisis represents a burst crisis resulting from highly complicated mortgage-backed securities, the unchecked distribution of mortgage loans, and a rapid speculative increase on the market surface. When analyzing the data of this period, numerous "anomalies" were evident, such as the disproportionate increase of housing prices relative to income, sharp rises in mortgage borrowing levels, and the decrease in rental yields compared to prices.
Had price predictions been made with deep learning derivatives such as LSTM during this period, deviations from trends could have served as early warning signals. Similarly, behavior trends such as "housing prices are skyrocketing," "everyone is buying houses," could have been highlighted through social media analysis or news sentiment analysis.
Additionally, the acceleration of interest in the subprime mortgage segment in the U.S. could have indicated the deterioration of credit portfolios through supervised learning models. By combining these signals, alarm levels could have been reached in specific markets before the 2008 crisis.
At this point, one might ask: If these models had truly existed in their current form at that time, would the crisis have been completely avoided? Perhaps not, but at least its impact could have been minimized, and damages could have been limited with tighter regulations and early policy actions.
The following types of data are critically important for bubble forecasting:
Time Series Forecasting: ARIMA, Prophet, LSTM
Anomaly Detection: Isolation Forest, One-Class SVM
Classification Models: XGBoost, Random Forest, Neural Networks
NLP and Social Data Analysis: BERT, sentiment analysis models
- Early warning systems for policymakers: Systems that notify central banks or municipalities of the market's overheating areas.
- Investor insights: Identifying areas with potential bubble signals for portfolio managers.
- Credit risk assessment: More careful evaluation of mortgages in risky areas for banks.
The real estate sector is rapidly evolving under the influence of digital transformation, and competition is increasing daily. In this dynamic environment, reaching the right customers and optimizing sales processes is critically important for success. PlusClouds' AI-supported lead generation tool, LeadOcean, provides significant advantages for real estate professionals in this area.
LeadOcean is an AI-based tool that analyzes your website to identify potential customers who are most aligned with the services you offer. It matches customer needs with the services you provide, identifying the most suitable customer candidates for you.
Smart Data Analysis: LeadOcean analyzes data available on the internet to identify potential customers that fit your industry. This way, you can focus on buyers who are genuinely interested.
Automated Customer Tracking: It helps you identify customers who are close to purchasing real estate by analyzing the behaviors of potential buyers.
Targeted Marketing: By reaching individuals who meet your specified criteria, it increases the efficiency of your advertising campaigns and allows you to reach the right people at the right time.
LeadOcean is a powerful tool that accelerates customer acquisition processes for professionals in the real estate sector, increases sales, and optimizes marketing strategies. With its AI-supported solutions, it helps you stand out in this competitive industry.
Artificial intelligence has immense potential in predicting anomalies and possible price bubbles in real estate markets. However, the goal of these systems should not be to create panic, but to provide timely and analytical information to decision-makers. In the future, such early warning systems may play a key role in maintaining financial stability.
Tracking the signs of crises can sometimes be the best way to prevent new ones. Artificial intelligence can illuminate this path for us.
إذا كنت صحفياً، لديك رغبة في التعمق في المواضيع المتعلقة بالأعمال والقطاعات، نريد العمل معك!
أثر الذكاء الاصطناعي على قطاع العقارات
Ece Kayaتكامل الذكاء الاصطناعي: 5 طرق لزيادة الكفاءة
Ece Kayaمدى استهلاك نماذج الذكاء الاصطناعي للطاقة؟ ما هو تناقض جيفونس؟
Ece Kayaسونيت كلود 3.7: عصر جديد في عالم الذكاء الاصطناعي
Ece Kayaالذكاء الاصطناعي، القطاع العام ومستقبل القوة العاملة: هل هو خطر أم فرصة؟
Ece KayaDeepSeek: مخاوف أمان البيانات، الحظر والمستقبل
Ece Kayaمع أداة AutoQuill التي بدأت PlusClouds بتقديمها لشركائها، سيتمكن منشئو المحتوى من البيع بنقرة واحدة فقط. دعنا نوضح لك كيف يمكنك الحصول على نصيبك من هذه الثورة.